Unlocking Incrementality: Ad Impact Measurement | Mobio Group
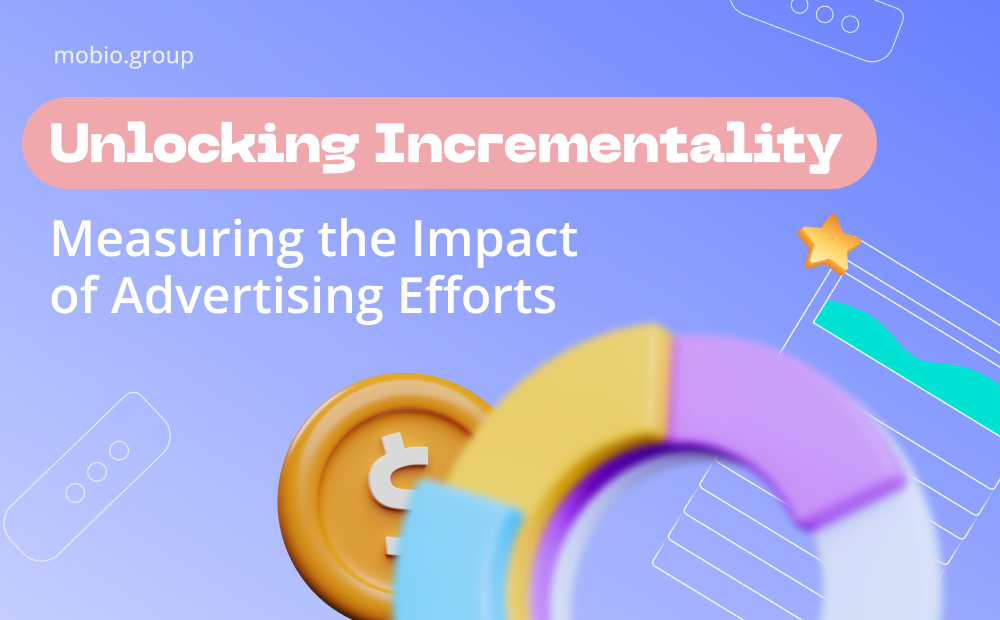
Paid advertising aims to generate additional revenue by increasing brand value and prompting actions such as purchases or app downloads. The key to evaluating advertising effectiveness is understanding which conversions are a direct result of advertising and which would have happened anyway. Measuring, attributing and analyzing marketing activity can be a complex task. In this article, Mobio Group will break down what incremental analysis is and what ways there are to measure the impact of an advertising campaign.
Understanding Incrementality in Marketing
Incrementality is the real added value created when certain actions lead to desired results. Specifically, when engaging mobile users, incrementality is a measure of the growth (e.g., number of installs or revenue) that results from investing in marketing efforts.
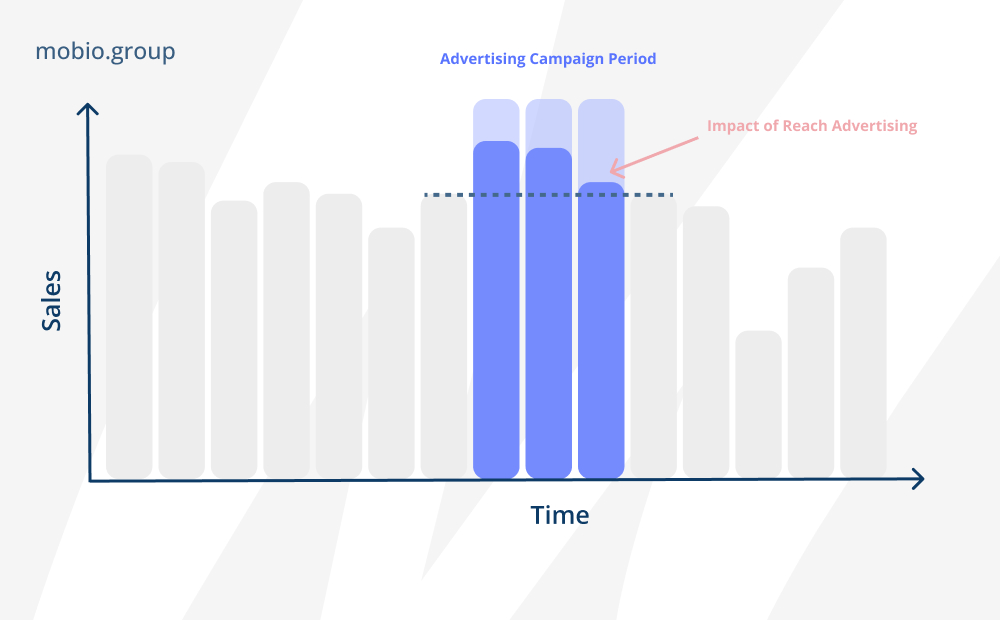
Incrementality research helps measure the true impact of advertising campaigns by answering key questions:
- How does advertising affect conversion rates?
- What is the real return on investment (ROI) of the advertising campaign?
- How did the brand’s marketing efforts affect market share?
- What happens if you increase or decrease the budget for channel X?
- On which channels is it better to optimize activity rates?
- How to correctly allocate the advertising budget?
The topic of incrementality is becoming increasingly popular due to some of the factors affecting marketing measurement:
⦿ Attribution issues
With the introduction of stricter privacy rules and data restrictions, attribution is becoming increasingly difficult, because for iOS users who do not share their IDFA, traditional attribution is no longer possible. Implementing SKAdNetwork for iOS attribution as a de facto solution provides limited information on long-term campaign performance, and this makes it difficult to accurately measure the impact of ad investments.
⦿ Distinguishing between paid and organic users
Advertisers face the challenge of separating paid and organic users who interact with their mobile apps. This is due to the labeling of non-IDFA users as “organic” in Mobile Measurement Partners (MMP) systems.
How the distinction between the concepts of attribution and incrementality is made, you can imagine by greatly narrowing down the amount of raw data. Let’s say you have some product X, which is successfully sold or brings stable conversions (100 per month). You decide to add product Y, run ads on it and attribute it. The chosen model will attribute a certain number of sales to product Y over an agreed period of time — 50. However, the incrementality of product Y will be less:
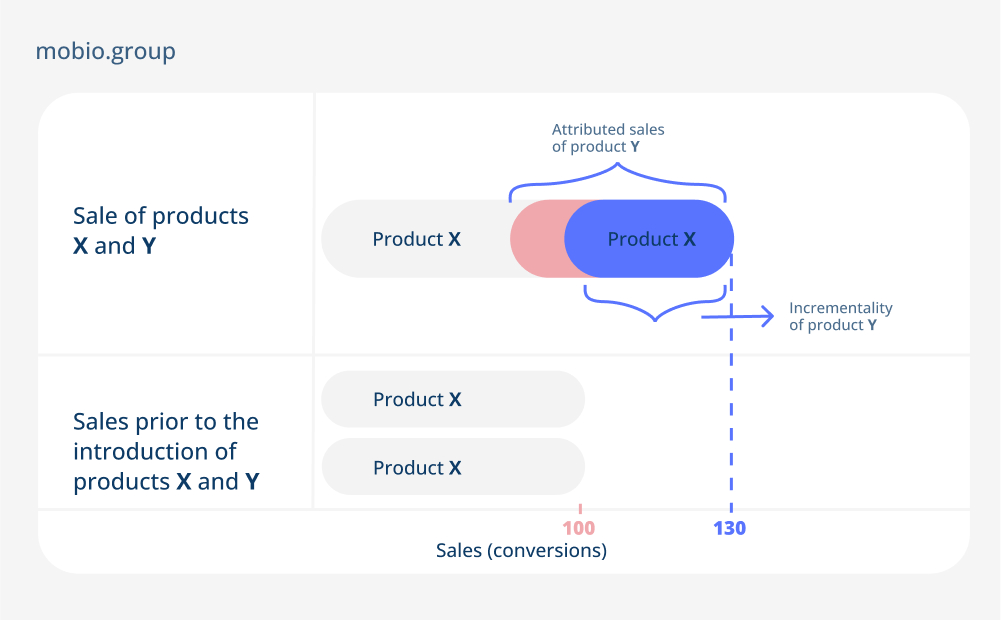
Despite its simplicity, this example quite clearly reflects the very essence of incrementality, which is inherent in its name — the added value of marketing activities. The only way to determine the impact of ad campaigns on the performance of almost anything, including cross-channel, WOM, offline and even non-media tactics, is to run incremental tests.
Incremental Analysis & Testing
Imagine this scenario: you allocate part of your budget to promoting YouTube ads, but later decide to stop because of the high cost of customer acquisition (CAC). Surprisingly, sales drop not only through YouTube, but also through direct views and organics. Even conversions from other marketing channels are down. This is the decline of the incremental effect.
So what is incremental testing? It is a controlled experiment that aims to distinguish between conversions caused by advertising campaigns and those that occur naturally (organic). For such a test, the main approach is A/B testing, which can be run on platforms such as Facebook, Instagram, Twitter, AppsFlyer or develop your own experiments.
Here’s how it works: Divide your audience into two groups, group A and group B, which exhibit the same behavior. Run campaigns for Group A only, and let Group B serve as a control group with no advertising. Since Group B conversions are completely organic, any increase seen in Group A can be attributed to the results of your marketing efforts. The simplest example:
Group A (experimental group, display advertising): 120 settings.
Group B (control group, no advertising): 100 installations.
Based on these numbers, we can calculate two important metrics: boost and increase. Uplift represents the increase of Group A compared to Group B (20 additional installations or an increase of 20%). Incrementality measures the percentage of conversions in Group A that can be attributed to the impact of marketing (20 installations is 16.7% of the total number of conversions in Group A). The higher the percentage of incrementality, the more effective the advertising campaign is.
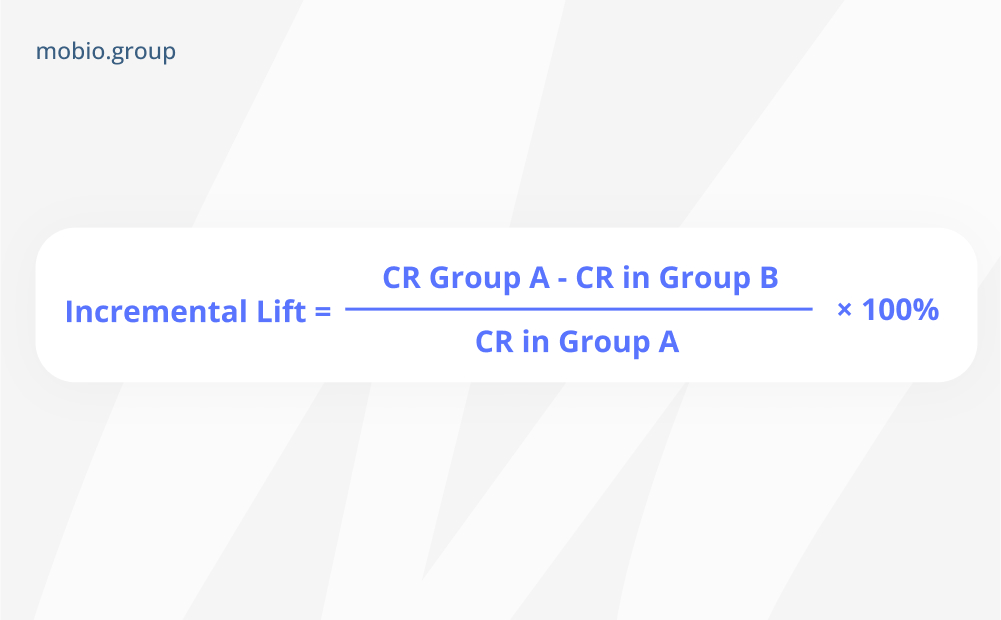
It is important to mention that the above example is simplified, and serves only as a starting point for understanding incremental analysis. More complex calculation models (e.g. regression models) take into account the influence of many external factors (seasonality, exchange rate fluctuations when calculating iROAS, etc.) and p-value for statistical calculations. It is important to be aware of the nuances of growth analysis beyond the simplified illustration and to consider their impact on the decrease (or increase) of the incremental effect.
To illustrate, let’s consider a subscription-based product with a low churn rate of about 15%. Suppose we launch a remarketing campaign targeting users who have 5 days left on their subscription, encouraging them to renew it. In this case, the incremental rate is expected to be low. Why? Because even without a remarketing campaign, most users are likely to renew their subscription anyway, as the Churn Rate is ~15%. This example demonstrates that a factor such as a “hot” audience can produce a lower % incremental rate at the expense of higher organic conversion rates.
The Bayesian method allows you to calculate even more accurately the probability that the desired user action was caused by exposure to advertising, but the calculation must delve into concepts such as a posteriori probability, beta and gamma distributions and incompatible hypotheses, which cannot be calculated by yourself in an Excel table (but you can try).
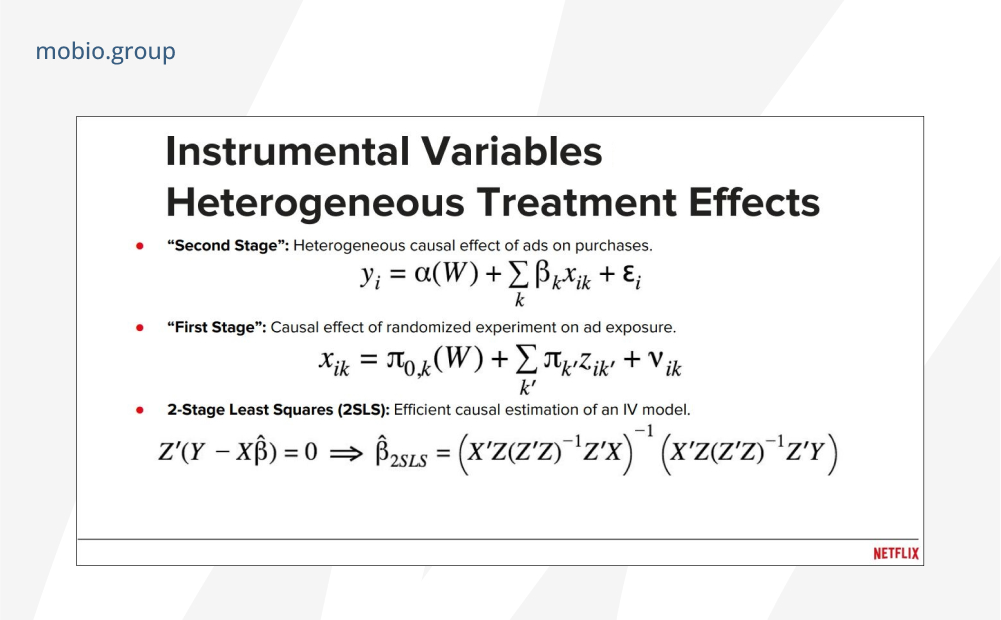
The evolution of the conversion funnel from a once simple click-to-purchase model to a complex and multi-channel landscape underscores the need for deep analytical approaches to capture and explore the multidimensional interactions between channels, audience segments and platforms on which brand ads are placed. Tools to support the technical part of conducting A/B tests with complex mathematical calculations are provided by data collection and analytics services and advertising platforms themselves.
Ways to Calculate Incrementality
Incremental testing, depending on the complexity and accuracy required, is carried out both with tools built into the advertising channel and independently. The main ways of assessing the impact of an advertising campaign:
⦿ A/B tests in a simple format
Classic A/B testing involves comparing users’ reactions to ads (the difference in perception by different audience segments or the impact of different ad creatives). Incremental tests show the difference in performance with advertising activity and with natural organics, as in the example with the experimental and control groups A and B.
⦿ Analysis of the correspondence
Analysis of the correspondence between the predicted patterns of conventional sales and the actual performance in an advertising campaign. A schematic example:
- measure the baseline
- forecast the trend
- adjust the forecast based on external factors (seasonal factor, product improvement, phase of the moon, etc.)
- compare the predicted results with the actual results after the advertising campaign.
⦿ Disabling the ad channel
This method is especially effective for trigger ads, where there is no lasting impact or residual effect on targeted actions. Not suitable if the traffic from organic sources is unstable. The technique involves stopping all marketing activities and establishing a baseline of conversions from organic sources. However, this method comes with some risks, especially when working with large volumes of paid traffic – completely shutting down the channel can potentially lead to a loss of income. In addition, this tactic does not exclude the influence of external factors such as seasonality, which can affect the final results.
⦿ Step-by-step launch of advertising channels
Conducted after measuring organic conversions or sales. By comparing the results of the staggered connection to the baseline, you can determine the effectiveness of each channel based on its growth. However, this method may not reflect the synergistic effect of marketing mix strategies, where multiple channels work together to increase overall effectiveness. Assessing the holistic impact of integrated marketing efforts requires a more comprehensive approach.
⦿ Abnormal scaling to an individual channel
By significantly increasing the advertising budget for a particular channel, you can observe the corresponding impact on other channels, their effectiveness, and the potential impact on the entire marketing ecosystem.
⦿ Brand lift
This is a built-in incremental test that is conducted on the ad site side. It focuses on measuring changes in users’ perception, awareness and attitudes toward the brand as a result of advertising efforts. The test assesses the impact of campaign launches on important brand metrics:
- Ad Recall — shows how well the target audience remembered the ad.
- Brand Awareness — assesses the impact of advertising on brand recognition and awareness among consumers.
- Purchase Intent — measures the increase in the percentage of users who are inclined to make a purchase after seeing an ad.
- Product Consideration — measures how often users choose a product after viewing an ad.
- Brand Favorability — examines how viewing an ad affects brand attitudes compared to competitors.
- Ad Message Recall — measures how effectively audiences remember a particular message conveyed in an ad.
Conducting Brand Lift research requires extensive surveys and data collection. It’s also an expensive way to do it — Facebook does this testing with the Experiments tool with minimum campaign budget requirements of $5k for Haiti and $30k for the US. Google also sets its own minimum budget requirements for Brand Lift research, depending on the country.
The Brand Lift method primarily focuses on brand-related metrics and does not provide a full understanding of other performance metrics such as sales, customer acquisition or ROI, even more so when influenced by parallel marketing campaigns and general market trends.
- Conversion-lift (AB-testing by actual actions). Such tests are conducted by advertising platforms to track additional conversions caused by a company’s marketing activities on the channel. This method is already becoming obsolete due to stricter privacy regulations. Facebook describes the testing process on its channel (specifying that no breakdowns by gender, age and country are provided for studies launched after July 13) as follows:
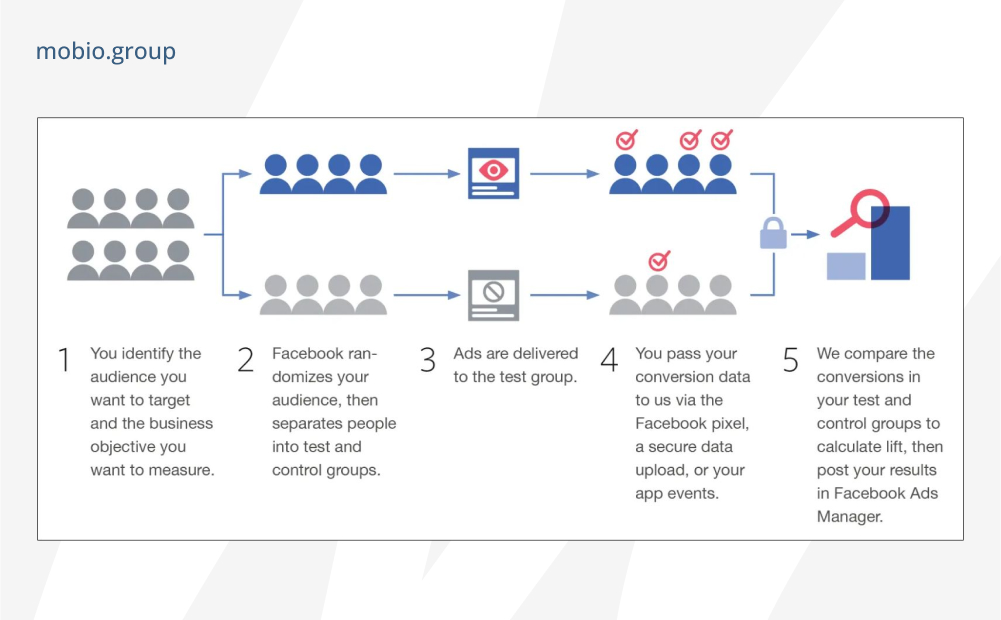
Conversion Lift also works for ads on YouTube and TrueView Discovery:
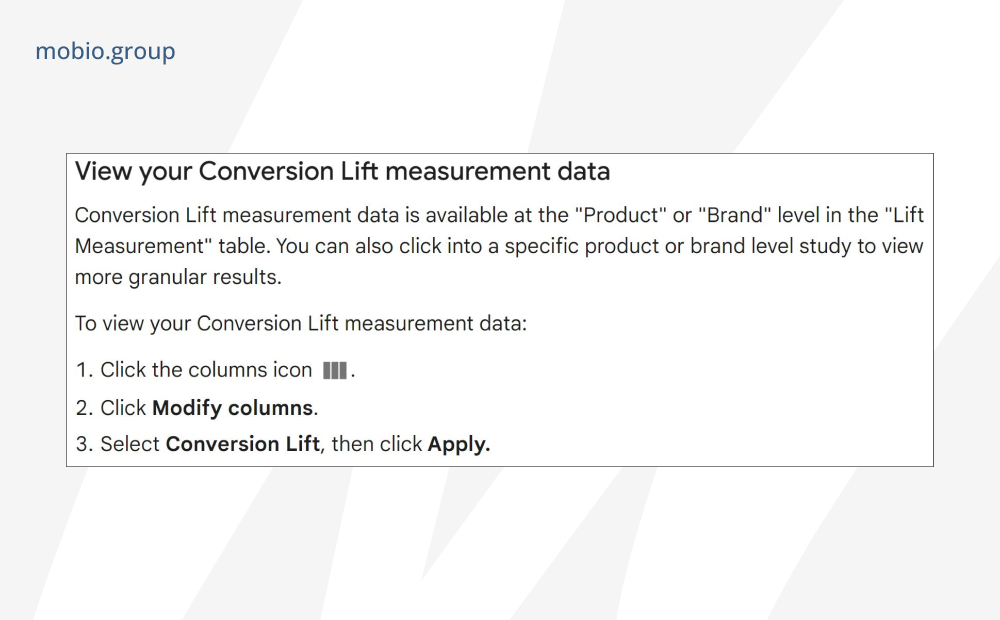
- incrementality measurement with Mobile Measurement Partners. AppsFlyer, for example, runs tests with such integrated partners:
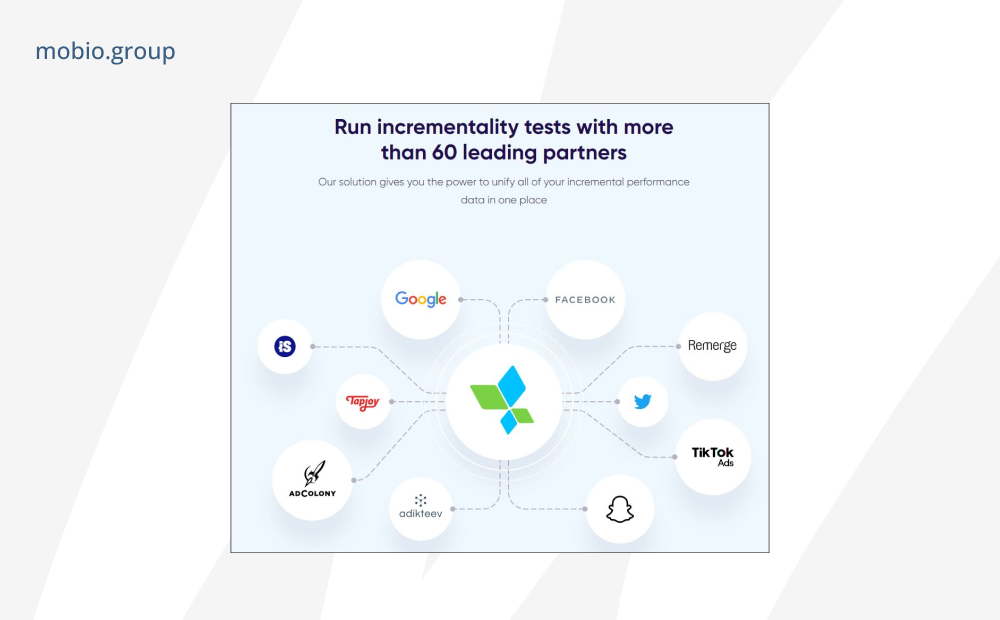
Factors Affecting Testing
To ensure accurate results in incremental tests, there are some factors to consider:
- Seasonality: Consider the timing of the tests and avoid conducting them during the peak season for your brand. Consistency in timing will help ensure accurate comparisons and prevent skewed results.
- Outliers and overlapping audiences: Maintain an unbiased control group, minimizing the influence of external factors such as other digital channels or media partners. Be cautious about changes to one part of the funnel that may overlap with testing of another, as this can affect the final results.
- Segment size and duration of testing: Adjust testing time depending on the specific circumstances of your business, ensuring statistical significance and avoiding jumping to conclusions. If your business attracts a large volume of traffic and generates many conversions, you can run tests with a smaller sample and still get statistically significant results. Conversely, if you don’t have a large business with fewer conversions, it’s recommended that you increase the length of your tests. With inline tests, platforms track themselves when the necessary data set is being collected.
- Short-term effects. During testing, you may see a temporary drop in results. However, this should be seen as an indication that your ads are working and that your marketing activities are affecting your results.
In recent years, changes in legislation and consumer privacy policies have created problems for tracking cookies and mobile identifiers, making it increasingly difficult to effectively track individual users. And it’s natural to wonder to what extent our advertising contributes to sales, revenue and overall business success, how product promotion would occur naturally without our intervention, and whether our investments in ASO, social media and other channels are really justified.
Incrementality offers a measurement approach that allows you to determine the impact of advertising tactics on key business performance indicators, not even relying on cookies or user-level data, but looking at the cause-and-effect relationships of advertising activity as a whole.
However, while the dimension of incrementality is more relevant than ever, there is some confusion around the concepts of incrementality and attribution, even though they have different meanings and serve different purposes. Attribution models focus on the distribution of conversion value across different touchpoints, offering insight into the customer journey, while incrementality allows us to delve deeper into the realm of cause and effect, revealing conversions that would not have occurred without our marketing tactics. In the next article, Mobio Group will take a closer look at the differences between these concepts, their advantages and disadvantages, and the impact of incremental tests on optimizing attribution models.